Artificial Neural Network (ANN) 5 - Checking gradient
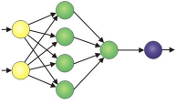
Continued from Artificial Neural Network (ANN) 4 - Back propagation where we computed the gradient of the cost function so that we are ready to train our Neural Network.
We're to test the gradient computation part of our code (sort of a unit test). It simply performs numerical gradient checking.
We'll use a simple quadratic function, $f(x)=x^2$
Then, we will compare two gradients:
$$f^\prime (x) = 2x$$with
$$ \frac {f(x+\Delta)-f(x-\Delta)}{2\Delta} $$The code and output are:
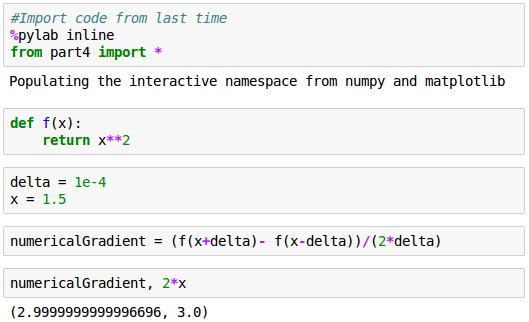
Let's add helper functions to our neural network class:
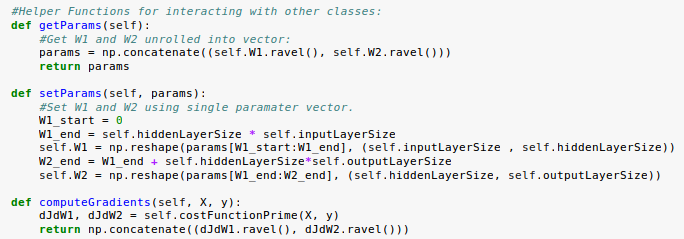
In getParams():
params = np.concatenate((self.W1.ravel(), self.W2.ravel()))
Given $W^{(1)}$ and $W^{(2)}$:
$$ W^{(1)} = \begin{bmatrix} W_{11}^{(1)} & W_{12}^{(1)} & W_{13}^{(1)} \\ W_{21}^{(1)} & W_{22}^{(1)} & W_{23}^{(1)} \end{bmatrix} $$ $$ W^{(2)} = \begin{bmatrix} W_{11}^{(2)} \\ W_{21}^{(2)} \\ W_{31}^{(2)} \end{bmatrix} $$The params becomes:
$$ params = \begin{bmatrix} W_{21}^{(1)} & W_{22}^{(1)} & W_{23}^{(1)} & W_{11}^{(1)} & W_{12}^{(1)} & W_{13}^{(1)} & W_{11}^{(2)} & W_{21}^{(2)} & W_{31}^{(2)} \end{bmatrix} $$We can use the same approach to numerically evaluate the gradient of our neural network.
However, it's a little more complicated this time since we have 9 gradient values, and we're interested in the gradient of our cost function.
We are going to make things simpler by testing one gradient at a time, and "perturb" each weight by adding epsilon to the current value and computing the cost function, subtracting epsilon from the current value and computing the cost function. Then, we compute the slope between these two values.
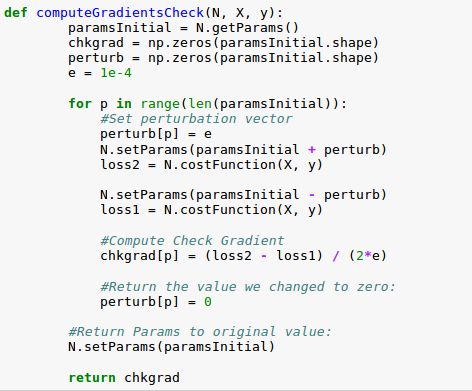
We repeat this process across all our weights, and when we're done we'll have a numerical gradient vector, with the same number of values as we have weights. It's this vector we would like to compare to our official gradient calculation.
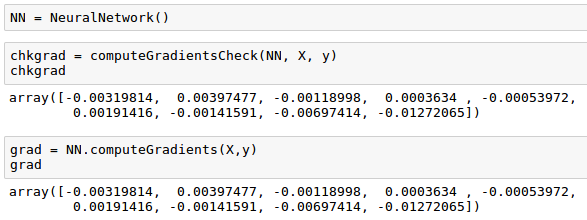
We see that our vectors appear very similar, which is a good sign, but we need to quantify just how similar they are by comparing the norms of the sum of the vectors:

Next:
6. Training via BFGSMachine Learning with scikit-learn
scikit-learn installation
scikit-learn : Features and feature extraction - iris dataset
scikit-learn : Machine Learning Quick Preview
scikit-learn : Data Preprocessing I - Missing / Categorical data
scikit-learn : Data Preprocessing II - Partitioning a dataset / Feature scaling / Feature Selection / Regularization
scikit-learn : Data Preprocessing III - Dimensionality reduction vis Sequential feature selection / Assessing feature importance via random forests
Data Compression via Dimensionality Reduction I - Principal component analysis (PCA)
scikit-learn : Data Compression via Dimensionality Reduction II - Linear Discriminant Analysis (LDA)
scikit-learn : Data Compression via Dimensionality Reduction III - Nonlinear mappings via kernel principal component (KPCA) analysis
scikit-learn : Logistic Regression, Overfitting & regularization
scikit-learn : Supervised Learning & Unsupervised Learning - e.g. Unsupervised PCA dimensionality reduction with iris dataset
scikit-learn : Unsupervised_Learning - KMeans clustering with iris dataset
scikit-learn : Linearly Separable Data - Linear Model & (Gaussian) radial basis function kernel (RBF kernel)
scikit-learn : Decision Tree Learning I - Entropy, Gini, and Information Gain
scikit-learn : Decision Tree Learning II - Constructing the Decision Tree
scikit-learn : Random Decision Forests Classification
scikit-learn : Support Vector Machines (SVM)
scikit-learn : Support Vector Machines (SVM) II
Flask with Embedded Machine Learning I : Serializing with pickle and DB setup
Flask with Embedded Machine Learning II : Basic Flask App
Flask with Embedded Machine Learning III : Embedding Classifier
Flask with Embedded Machine Learning IV : Deploy
Flask with Embedded Machine Learning V : Updating the classifier
scikit-learn : Sample of a spam comment filter using SVM - classifying a good one or a bad one
Machine learning algorithms and concepts
Batch gradient descent algorithmSingle Layer Neural Network - Perceptron model on the Iris dataset using Heaviside step activation function
Batch gradient descent versus stochastic gradient descent
Single Layer Neural Network - Adaptive Linear Neuron using linear (identity) activation function with batch gradient descent method
Single Layer Neural Network : Adaptive Linear Neuron using linear (identity) activation function with stochastic gradient descent (SGD)
Logistic Regression
VC (Vapnik-Chervonenkis) Dimension and Shatter
Bias-variance tradeoff
Maximum Likelihood Estimation (MLE)
Neural Networks with backpropagation for XOR using one hidden layer
minHash
tf-idf weight
Natural Language Processing (NLP): Sentiment Analysis I (IMDb & bag-of-words)
Natural Language Processing (NLP): Sentiment Analysis II (tokenization, stemming, and stop words)
Natural Language Processing (NLP): Sentiment Analysis III (training & cross validation)
Natural Language Processing (NLP): Sentiment Analysis IV (out-of-core)
Locality-Sensitive Hashing (LSH) using Cosine Distance (Cosine Similarity)
Artificial Neural Networks (ANN)
[Note] Sources are available at Github - Jupyter notebook files1. Introduction
2. Forward Propagation
3. Gradient Descent
4. Backpropagation of Errors
5. Checking gradient
6. Training via BFGS
7. Overfitting & Regularization
8. Deep Learning I : Image Recognition (Image uploading)
9. Deep Learning II : Image Recognition (Image classification)
10 - Deep Learning III : Deep Learning III : Theano, TensorFlow, and Keras
Ph.D. / Golden Gate Ave, San Francisco / Seoul National Univ / Carnegie Mellon / UC Berkeley / DevOps / Deep Learning / Visualization