scikit-learn : Bias-variance tradeoff

The bias-variance tradeoff is a central problem in supervised learning.
Ideally, one wants to choose a model that both accurately captures the regularities in its training data, but also generalizes well to unseen data.
Unfortunately, it is typically impossible to do both simultaneously.
High-variance learning methods may be able to represent their training set well, but are at risk of overfitting to noisy or unrepresentative training data.
In contrast, algorithms with high bias typically produce simpler models that don't tend to overfit, but may underfit their training data, failing to capture important regularities.
Source : Bias-variance tradeoff
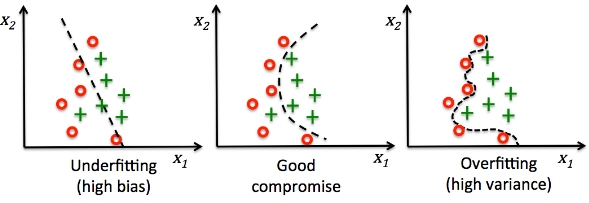
picture source: Python Machine Learning - Sebastian Raschka
picture source: Understanding the Bias-Variance Tradeoff
Suppose we want to approximate a $\sin {\pi x}$ function with two points ($N=2$).
We're going to use two sets of hypothesis , $\mathcal H_0$ and $\mathcal H_1$.
$\mathcal H_0$ is a hypothesis of using a const ($y=C$) while $\mathcal H_1$ using a line ($y=ax+b$).
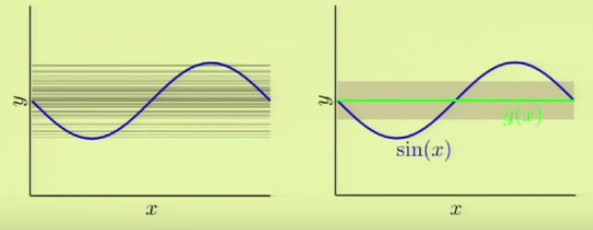
The left figure shows outputs from const hypothesis($\mathcal H_0$), and each line is approximation corresponding each data set. The picture on the right side shows the the averaged target hypothesis ($\bar g(x)$) we get and the gray strip tells us the variance. Note that for each learning, we get one of the lines in the picture below, and the green avareged hypothesis $\bar g(x)$ is actually the best approximation we get.
Now, we want to play with $\mathcal H_1$ with the same data set we used in the previous picture. The output is shown in the figure below:
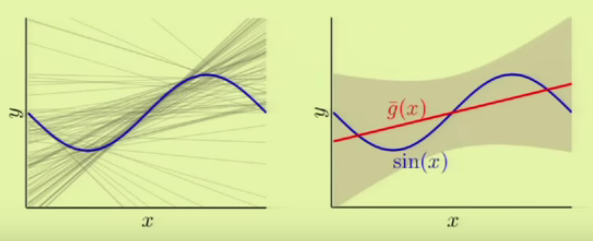
It appears we now have better approximation in terms of averaged hypothesis ($\bar g(x)$) while we got greater variance.
Let's compare the two side by side:
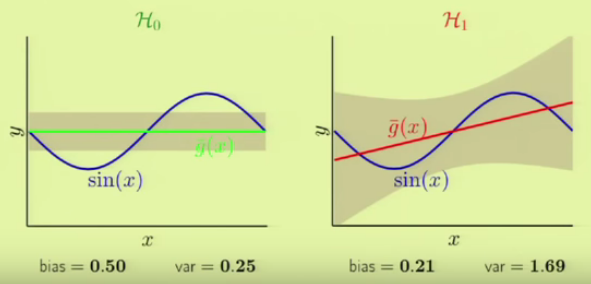
Picture source: Caltech : Lecture 08 - Bias-Variance Tradeoff
At its root, dealing with bias and variance is really about dealing with over- and under-fitting.
Bias is reduced and variance is increased in relation to model complexity. As more and more parameters are added to a model, the complexity of the model rises and variance becomes our primary concern while bias steadily falls.
For example, as more polynomial terms are added to a linear regression, the greater the resulting model's complexity will be.
![]()
Understanding bias and variance is critical for understanding the behavior of prediction models, but in general what we really care about is overall error, not the specific decomposition.
The sweet spot for any model is the level of complexity at which the decrease in bias is equivalent to the increase in variance. - ref. Understanding the Bias-Variance Tradeoff
Machine Learning with scikit-learn
scikit-learn installation
scikit-learn : Features and feature extraction - iris dataset
scikit-learn : Machine Learning Quick Preview
scikit-learn : Data Preprocessing I - Missing / Categorical data
scikit-learn : Data Preprocessing II - Partitioning a dataset / Feature scaling / Feature Selection / Regularization
scikit-learn : Data Preprocessing III - Dimensionality reduction vis Sequential feature selection / Assessing feature importance via random forests
Data Compression via Dimensionality Reduction I - Principal component analysis (PCA)
scikit-learn : Data Compression via Dimensionality Reduction II - Linear Discriminant Analysis (LDA)
scikit-learn : Data Compression via Dimensionality Reduction III - Nonlinear mappings via kernel principal component (KPCA) analysis
scikit-learn : Logistic Regression, Overfitting & regularization
scikit-learn : Supervised Learning & Unsupervised Learning - e.g. Unsupervised PCA dimensionality reduction with iris dataset
scikit-learn : Unsupervised_Learning - KMeans clustering with iris dataset
scikit-learn : Linearly Separable Data - Linear Model & (Gaussian) radial basis function kernel (RBF kernel)
scikit-learn : Decision Tree Learning I - Entropy, Gini, and Information Gain
scikit-learn : Decision Tree Learning II - Constructing the Decision Tree
scikit-learn : Random Decision Forests Classification
scikit-learn : Support Vector Machines (SVM)
scikit-learn : Support Vector Machines (SVM) II
Flask with Embedded Machine Learning I : Serializing with pickle and DB setup
Flask with Embedded Machine Learning II : Basic Flask App
Flask with Embedded Machine Learning III : Embedding Classifier
Flask with Embedded Machine Learning IV : Deploy
Flask with Embedded Machine Learning V : Updating the classifier
scikit-learn : Sample of a spam comment filter using SVM - classifying a good one or a bad one
Machine learning algorithms and concepts
Batch gradient descent algorithmSingle Layer Neural Network - Perceptron model on the Iris dataset using Heaviside step activation function
Batch gradient descent versus stochastic gradient descent
Single Layer Neural Network - Adaptive Linear Neuron using linear (identity) activation function with batch gradient descent method
Single Layer Neural Network : Adaptive Linear Neuron using linear (identity) activation function with stochastic gradient descent (SGD)
Logistic Regression
VC (Vapnik-Chervonenkis) Dimension and Shatter
Bias-variance tradeoff
Maximum Likelihood Estimation (MLE)
Neural Networks with backpropagation for XOR using one hidden layer
minHash
tf-idf weight
Natural Language Processing (NLP): Sentiment Analysis I (IMDb & bag-of-words)
Natural Language Processing (NLP): Sentiment Analysis II (tokenization, stemming, and stop words)
Natural Language Processing (NLP): Sentiment Analysis III (training & cross validation)
Natural Language Processing (NLP): Sentiment Analysis IV (out-of-core)
Locality-Sensitive Hashing (LSH) using Cosine Distance (Cosine Similarity)
Artificial Neural Networks (ANN)
[Note] Sources are available at Github - Jupyter notebook files1. Introduction
2. Forward Propagation
3. Gradient Descent
4. Backpropagation of Errors
5. Checking gradient
6. Training via BFGS
7. Overfitting & Regularization
8. Deep Learning I : Image Recognition (Image uploading)
9. Deep Learning II : Image Recognition (Image classification)
10 - Deep Learning III : Deep Learning III : Theano, TensorFlow, and Keras
Ph.D. / Golden Gate Ave, San Francisco / Seoul National Univ / Carnegie Mellon / UC Berkeley / DevOps / Deep Learning / Visualization