scikit-learn : Decision Tree Learning II - Constructing the Decision Tree

Continued from scikit-learn : Decision Tree Learning I - Entropy, Gini, and Information Gain
In this article, we'll build a decision tree using Information Gain (IG).
Decision Tree.Though decision trees can construct complex decision boundaries by dividing the feature space into rectangles, we should be careful not to make deep decision tree since it may easily result in overfitting.
We construct the tree as follows:
- First, we choose an attribute A with highest information gain for the full training set at the root of the tree.
- Construct child nodes for each value of A. Each has an associated subset of vectors in which A has a particular value.
We will use scikit-learn to build a decision tree with a maximum depth of 3 using entropy as a criterion for impurity.
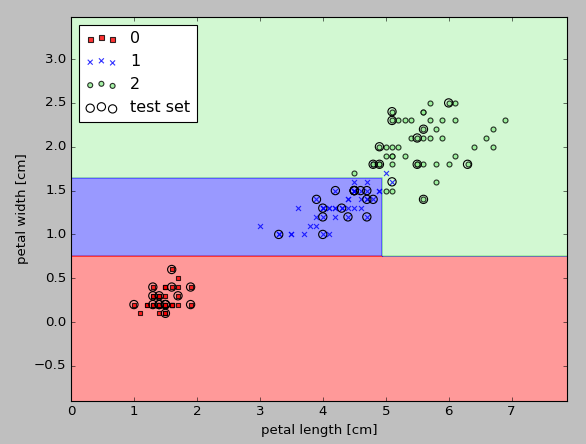
Here is the code for the plot:
from sklearn import datasets from sklearn.cross_validation import train_test_split from sklearn.tree import DecisionTreeClassifier import numpy as np import matplotlib.pyplot as plt from matplotlib.colors import ListedColormap iris = datasets.load_iris() X = iris.data[:, [2, 3]] y = iris.target X_train, X_test, y_train, y_test = train_test_split(X, y, test_size=0.3, random_state=0) tree = DecisionTreeClassifier(criterion='entropy', max_depth=3, random_state=0) tree.fit(X_train, y_train) X_combined = np.vstack((X_train, X_test)) y_combined = np.hstack((y_train, y_test)) def plot_decision_regions(X, y, classifier, test_idx=None, resolution=0.02): # setup marker generator and color map markers = ('s', 'x', 'o', '^', 'v') colors = ('red', 'blue', 'lightgreen', 'gray', 'cyan') cmap = ListedColormap(colors[:len(np.unique(y))]) # plot the decision surface x1_min, x1_max = X[:, 0].min() - 1, X[:, 0].max() + 1 x2_min, x2_max = X[:, 1].min() - 1, X[:, 1].max() + 1 xx1, xx2 = np.meshgrid(np.arange(x1_min, x1_max, resolution), np.arange(x2_min, x2_max, resolution)) Z = classifier.predict(np.array([xx1.ravel(), xx2.ravel()]).T) Z = Z.reshape(xx1.shape) plt.contourf(xx1, xx2, Z, alpha=0.4, cmap=cmap) plt.xlim(xx1.min(), xx1.max()) plt.ylim(xx2.min(), xx2.max()) # plot all samples X_test, y_test = X[test_idx, :], y[test_idx] for idx, cl in enumerate(np.unique(y)): plt.scatter(x=X[y == cl, 0], y=X[y == cl, 1], alpha=0.8, c=cmap(idx), marker=markers[idx], label=cl) # highlight test samples if test_idx: X_test, y_test = X[test_idx, :], y[test_idx] plt.scatter(X_test[:, 0], X_test[:, 1], c='', alpha=1.0, linewidth=1, marker='o', s=55, label='test set') plot_decision_regions(X_combined, y_combined, classifier=tree, test_idx=range(105,150)) plt.xlabel('petal length [cm]') plt.ylabel('petal width [cm]') plt.legend(loc='upper left') plt.show() from sklearn.tree import export_graphviz export_graphviz(tree, out_file='tree.dot', feature_names=['petal length', 'petal width'])
Scikit-learn allows us to export the decision tree as a .dot file after training as shown in the code of the previous section.
Using GraphViz we can visualize the tree.
We can install graphviz-dev package on Ubuntu 16.04:
$ sudo apt-get update $ sudo apt-get install graphviz-dev $ sudo apt-get install graphviz
After installing GraphViz, we can convert the tree.dot file into a PNG file:
$ dot -Tpng tree.dot -o tree.png
Here is th decision tree created via GraphViz:
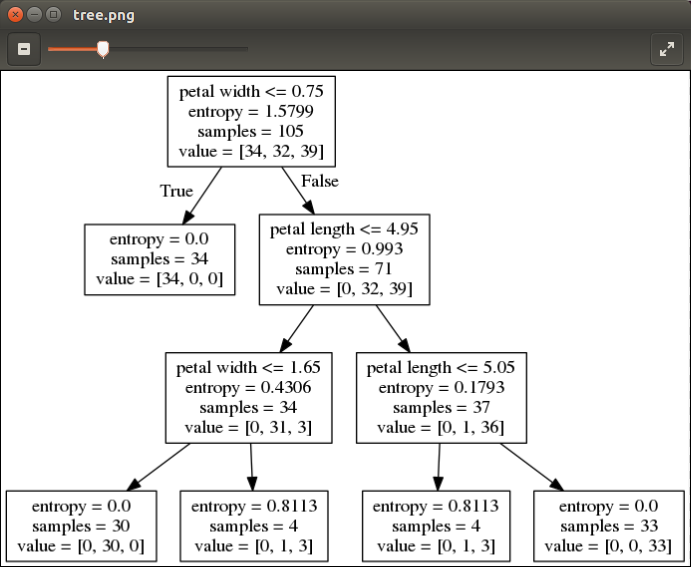
From the picture above, we can trace back the splits by the decision tree from our training dataset.
Starting with 105 samples at the root, we split it into two child nodes with 34 and 71 samples each using the petal with $cut-off \le 0.75 cm$.
After the first split, we can see that the left child node is already pure and only contains samples from the Iris-Setosa class (entropy = 0).
On the right side, we separate the samples from the Iris-Versicolor and Iris-Virginica classes.
Ref: Python Machine Learing by Sebastian Raschka
Machine Learning with scikit-learn
scikit-learn installation
scikit-learn : Features and feature extraction - iris dataset
scikit-learn : Machine Learning Quick Preview
scikit-learn : Data Preprocessing I - Missing / Categorical data
scikit-learn : Data Preprocessing II - Partitioning a dataset / Feature scaling / Feature Selection / Regularization
scikit-learn : Data Preprocessing III - Dimensionality reduction vis Sequential feature selection / Assessing feature importance via random forests
Data Compression via Dimensionality Reduction I - Principal component analysis (PCA)
scikit-learn : Data Compression via Dimensionality Reduction II - Linear Discriminant Analysis (LDA)
scikit-learn : Data Compression via Dimensionality Reduction III - Nonlinear mappings via kernel principal component (KPCA) analysis
scikit-learn : Logistic Regression, Overfitting & regularization
scikit-learn : Supervised Learning & Unsupervised Learning - e.g. Unsupervised PCA dimensionality reduction with iris dataset
scikit-learn : Unsupervised_Learning - KMeans clustering with iris dataset
scikit-learn : Linearly Separable Data - Linear Model & (Gaussian) radial basis function kernel (RBF kernel)
scikit-learn : Decision Tree Learning I - Entropy, Gini, and Information Gain
scikit-learn : Decision Tree Learning II - Constructing the Decision Tree
scikit-learn : Random Decision Forests Classification
scikit-learn : Support Vector Machines (SVM)
scikit-learn : Support Vector Machines (SVM) II
Flask with Embedded Machine Learning I : Serializing with pickle and DB setup
Flask with Embedded Machine Learning II : Basic Flask App
Flask with Embedded Machine Learning III : Embedding Classifier
Flask with Embedded Machine Learning IV : Deploy
Flask with Embedded Machine Learning V : Updating the classifier
scikit-learn : Sample of a spam comment filter using SVM - classifying a good one or a bad one
Machine learning algorithms and concepts
Batch gradient descent algorithmSingle Layer Neural Network - Perceptron model on the Iris dataset using Heaviside step activation function
Batch gradient descent versus stochastic gradient descent
Single Layer Neural Network - Adaptive Linear Neuron using linear (identity) activation function with batch gradient descent method
Single Layer Neural Network : Adaptive Linear Neuron using linear (identity) activation function with stochastic gradient descent (SGD)
Logistic Regression
VC (Vapnik-Chervonenkis) Dimension and Shatter
Bias-variance tradeoff
Maximum Likelihood Estimation (MLE)
Neural Networks with backpropagation for XOR using one hidden layer
minHash
tf-idf weight
Natural Language Processing (NLP): Sentiment Analysis I (IMDb & bag-of-words)
Natural Language Processing (NLP): Sentiment Analysis II (tokenization, stemming, and stop words)
Natural Language Processing (NLP): Sentiment Analysis III (training & cross validation)
Natural Language Processing (NLP): Sentiment Analysis IV (out-of-core)
Locality-Sensitive Hashing (LSH) using Cosine Distance (Cosine Similarity)
Artificial Neural Networks (ANN)
[Note] Sources are available at Github - Jupyter notebook files1. Introduction
2. Forward Propagation
3. Gradient Descent
4. Backpropagation of Errors
5. Checking gradient
6. Training via BFGS
7. Overfitting & Regularization
8. Deep Learning I : Image Recognition (Image uploading)
9. Deep Learning II : Image Recognition (Image classification)
10 - Deep Learning III : Deep Learning III : Theano, TensorFlow, and Keras
Ph.D. / Golden Gate Ave, San Francisco / Seoul National Univ / Carnegie Mellon / UC Berkeley / DevOps / Deep Learning / Visualization